Google Analytics boss: "Generative AI will finally give us access to all data"
Gerrit Kazmaier is responsible for everything to do with data analysis at Google Cloud. In this interview, he explains what this means in the age of AI.
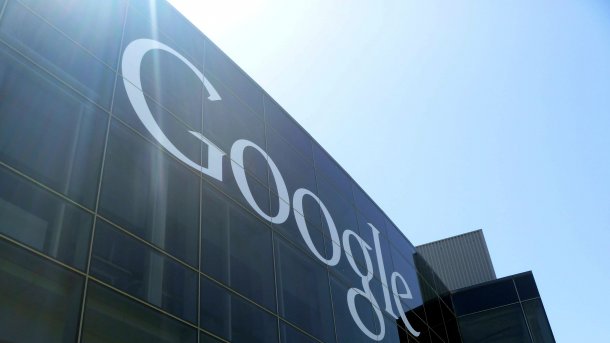
(Bild: Daniel AJ Sokolov)
Gerrit Kazmeier holds the title of Vice President and General Manager for Data and Analytics at Google Cloud. Before moving to Silicon Valley, he was President of the HANA and Analytics team at SAP in Germany, where he was responsible for databases and data warehousing. His work at SAP also took him to Vancouver, Canada, as Vice President of SAP Analytics Cloud. He studied in Constance and Nottingham.
When people think of Google Cloud, they often think of BigQuery, your data warehouse system. How did it come about?
BigQuery was originally invented to solve Google's own data processing challenges. Our data volumes quickly became enormous. Trillions of individual data records had to be aggregated very dynamically - just for Google AdSense and the rest of the advertising business. Because there was no off-the-shelf solution that could scale sufficiently and manage this, Google then built it themselves, using our engineering talent.
BigQuery was then quickly opened up to customers.
Yes, it is one of the most widely used systems. Our customers, there are now over 10,000 of them, make around a trillion data queries a day on BigQuery - and that's all without Google itself. We now call it an AI-ready data foundation that accomplishes many things that are incredibly important for the successful application of AI.
First of all, AI needs training data.
Precisely in order to learn anything meaningful at all. And there are, of course, incredibly powerful foundation models that are trained on general public data and can do amazing things. But of course they don't know anything about what's in a specific company index. For us, this leads to the important realization that the data strategy and the AI strategy are actually almost one and the same, two sides of the same coin. In addition, analytics, i.e. data analysis and my second job, is of course increasingly taking place through AI itself.
Company data is often like a needle in a haystack because it is unstructured.
And there is more and more of it. In addition to office documents, there are more and more videos, images and audio files, all things that are not typically part of an enterprise data landscape because it was simply too difficult to analyze them until now. With generative AI, all of this suddenly becomes very dynamic and can be read in as flexibly as an SQL table that can be easily queried.
But what can you do with it in practice?
You can do the most insane things with it. For example, if I want to know how customers feel about my product, how high the risk is that I could lose them. You can then analyze sound files from support and draw conclusions from them. That's a real treasure trove. Or take communication with suppliers or everything in emails and social media posts. That represents incredible value if you can analyze it. And this is finally possible with generative AI; we can now really get our hands on this data.
Google is known for processing a wide variety of data, be it text, images, videos or audio. How helpful is this for corporate customers?
First of all, the multimodality of the data is a very important feature that BigQuery can handle. The other thing we can do is connect directly to Vertex AI, our unified AI platform. This means that if I have my data in BigQuery, documents for example, and I now want to use the Document API, I can simply say programmatically in BigQuery with Python: Hey, connect this data, this table with this Large Language Model and extract this and that for me. And then BigQuery does this in the background without you having to export or integrate data yourself.
Google is data-driven. Some cloud customers are probably afraid that their own data will be used for training.
Of course, none of it reaches Google. Everything is strictly separated. There is a strict firewall. Irrespective of the basic guarantees that every customer has, they can of course also use their own encryption, determine how data is stored, secure data in processing in the same way as data in transit.
Google Cloud has numerous large customers, including Apple, who use their servers for iCloud - in addition to Amazon Web Services and their own infrastructure.
Here too, customer data is customer data.
Like all AI providers, Google has the problem that large language models are still hallucinating - and users do not know what is true and what is not true from the text output. This is a problem with business data.
It's incredibly important to do sufficient grounding - or, as we say in German, to "ground" the models on a factual basis. We provide a range of services in Vertex AI and BigQuery that do just that.
How exactly? Is Google search included?
Search, of course, but also via special ways of querying the model, via special checks. This affects the entire lifecycle, not just the prompt. It starts with how the models are trained. We need error detection.
If the model then says: I think this metric is interesting, then we can, of course, say, please match it with the available data to determine whether it is based on facts. If it's not, you give it back to the model and try again. So there are many strategies that reduce this problem of hallucinations as much as possible. And ultimately, we always end up back at the hard data.
Google Cloud gives its customers access to a Model Garden via Vertex AI, which also contains competing systems to Gemini. "Discover and use the widest possible variety of model types," writes Google. Should this be maintained?
Absolutely.
If Google were to sign a contract with OpenAI now, would GPT-4 be included?
That's a good question, and I'm probably the wrong person to answer it. (laughs) But I think our principles are clear. We offer all the models we can offer - our own foundation models, other first-party models and also resource models. And the idea behind it is that all models have different characteristics in terms of cost, performance, latency or specialization for a certain use case. And it is crucial for our customers to be able to select the right model for the proper context.
Because one thing is clear: we are talking about a whole new world or a whole new mindset here because much of what we have done so far is changing completely. Data analytics was once made for people, for static applications, for dashboards, KPIs and so on. Now we live in a world where we say data analytics will probably be used massively by new intelligent agents this year and in the following years. This is a whole new paradigm. With generative AI, we finally have access to all the data.
What does this do to companies?
Two years ago, it would likely have been said that they were all ultimately software companies. They all hired developers, they all learned what a software lifecycle is, what a service is, whether it was a washing machine manufacturer or a maintenance company.
And now, I think you're getting to the point where every company is actually saying, well, we're actually an AI company to a large extent because we have a unique asset. And that is our data, which describes our company. All our intellectual property that makes us unique is in there. And now we need to use the right AI models to activate this data that makes us so special.
The interview has been edited and shortened for better readability. The author traveled to Google Cloud Next at the invitation of Google.
(bsc)